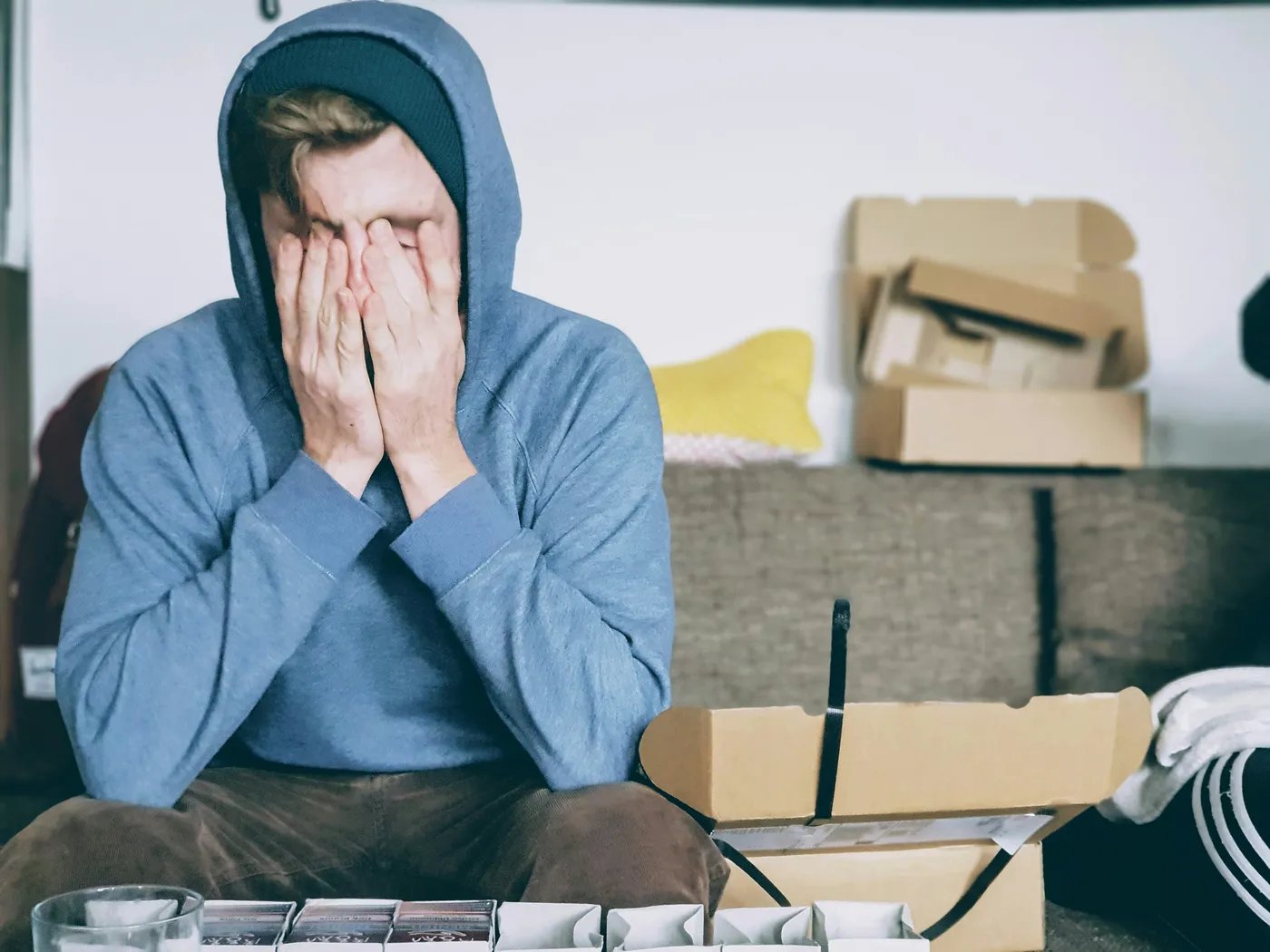
Making Sense of AI Tools: A No-Nonsense Guide for Busy Professionals
Last week, I sat down with my coffee and opened my inbox to find 17 emails about new AI tools. Sound familiar? From LinkedIn posts about the "next revolutionary AI platform" to Slack channels buzzing with the latest tool recommendations, the noise is deafening. My clients, ranging from solo entrepreneurs to Fortune 500 executives, all share the same challenge: how do we cut through the hype and find tools that actually deliver value?
The Reality Check: Why We're All Overwhelmed 🌐
Remember when choosing software meant picking between Microsoft Office and OpenOffice? Those days are long gone. Today's landscape is a maze of options, each promising to transform your workflow. During a recent workshop with tech leaders, we mapped out over 200 AI tools launched in just the last quarter. From content creation to visual AI, and development tools to productivity automation, the options seem endless. It's not just about quantity – it's about the pace of change. Tools that seemed cutting-edge last month might already be outdated.
A Framework That Actually Works 🎯
Through hundreds of consultations and implementations, I've developed a battle-tested approach to evaluating AI tools. Here's what matters:
1. Define Your Core Needs First
Before you even look at tools, answer these questions:
- What specific problem keeps you up at night?
- Which tasks eat up most of your team's time?
- What's your realistic budget and learning curve tolerance?
Pro tip: Write these answers down. You'll be surprised how many shiny features become irrelevant when you focus on your core needs.
2. Security and Privacy Checkpoints ⚔️
In my experience auditing AI implementations, security often gets overlooked in the excitement of new capabilities. Essential checks include:
- How is your data handled and stored?
- What's their track record with security incidents?
- Do they meet your industry's compliance requirements?
- Can you easily export or delete your data?
3. Integration and Scalability 🔄
I recently watched a company waste six months on an AI tool that couldn't integrate with their existing stack. Learn from their mistake. Verify:
- API reliability and documentation quality
- Compatibility with your current tools
- Growth capacity without performance degradation
- Data portability options
4. Community and Support Structure
A thriving community often signals a tool's longevity. Look for:
- Active discussion forums (not just marketing noise)
- Regular feature updates and bug fixes
- Responsive support teams
- Clear documentation and tutorials
Red Flags from the Trenches 🚩
After reviewing hundreds of AI tools, these warning signs consistently predict problems:
- Vague data handling policies
- Hidden pricing tiers
- Missing company information
- Poor documentation
- Aggressive data access requirements
Making the Final Decision: A Practical Approach
Start with a pilot project. Last month, I guided a marketing team through this process:
- Two-Week Test: They tried three tools with a small team
- Data Collection: Tracked specific metrics and user feedback
- Team Input: Gathered insights from daily users
- Performance Review: Measured against predetermined KPIs
For example, when evaluating AI writing tools, the team focused on output quality and integration with their existing CMS. For image generation, they prioritized style consistency and batch processing capabilities.
Future-Proofing Your Choice 🔮
The AI landscape shifts weekly. Choose tools that demonstrate:
- Consistent innovation without stability issues
- Clear communication about updates and roadmap
- Strong financial backing or sustainable business model
- Active development and community engagement
Making Peace with Imperfection
Here's the truth: perfect AI tools don't exist. After implementing hundreds of solutions, I've learned to focus on finding the right balance between:
- Must-have features and nice-to-haves
- Current needs and future potential
- Security requirements and usability
- Cost and expected ROI
Looking Ahead: Your Next Steps
- Start Small: Choose one pressing problem to solve
- Document Your Journey: Track what works and what doesn't
- Stay Flexible: Be ready to pivot if needs change
- Build Internal Knowledge: Train your team gradually
Remember: The goal isn't to have the most advanced AI stack – it's to solve real business problems effectively. Check out our detailed reviews to see how different tools stack up against these criteria.